In this article, we discuss how to promote machine learning in finance. Using this material, you’ll find out about the key benefits and use cases.
Machine learning in finance offers massive long-term prospects. The financial sector is among the most lucrative fields in modern business. Why? It’s impossible to live without the use of personal finance. A person who doesn’t have a bank account and credit card is unlikely to have a comfortable life in daily scenarios. While cash payments are still available in many places, the trend toward their removal is evident. Internet transactions, for example, are almost impossible without modern finance technology. In this article, we look at the aspects that can make the lives of people using financial services easier. What is it? We want to discuss the issue of machine learning in finance. As you’ll see, frameworks such as AI can revolutionize the current approaches used by banks and financial organizations.
What is ML in Finance?
One of the key factors in finance is the presence of a tremendous amount of data. The users perform millions of transactions daily, providing strong insights into their behavior. In the past, banks and financial organizations had limited capability to analyze this information. They could find general trends in the data, with the advanced details requiring too much computational power. In this regard, machine learning in finance solves the problem once and for all. Machines aren’t constrained regarding data analysis to the extent that humans are. They can review tremendous volumes of information fast and without getting tired. What does this offer for finance? By reviewing the information about the transactions, machine learning tools present high-quality statistics about even the most minute phenomena. One can learn not only the overall purchase preferences of the population but also create individual customer profiles and even deduce when certain products sell the best. In short, machine learning helps make financial data analysis as targeted as possible.
The Significance of Machine Learning for the Finance Industry
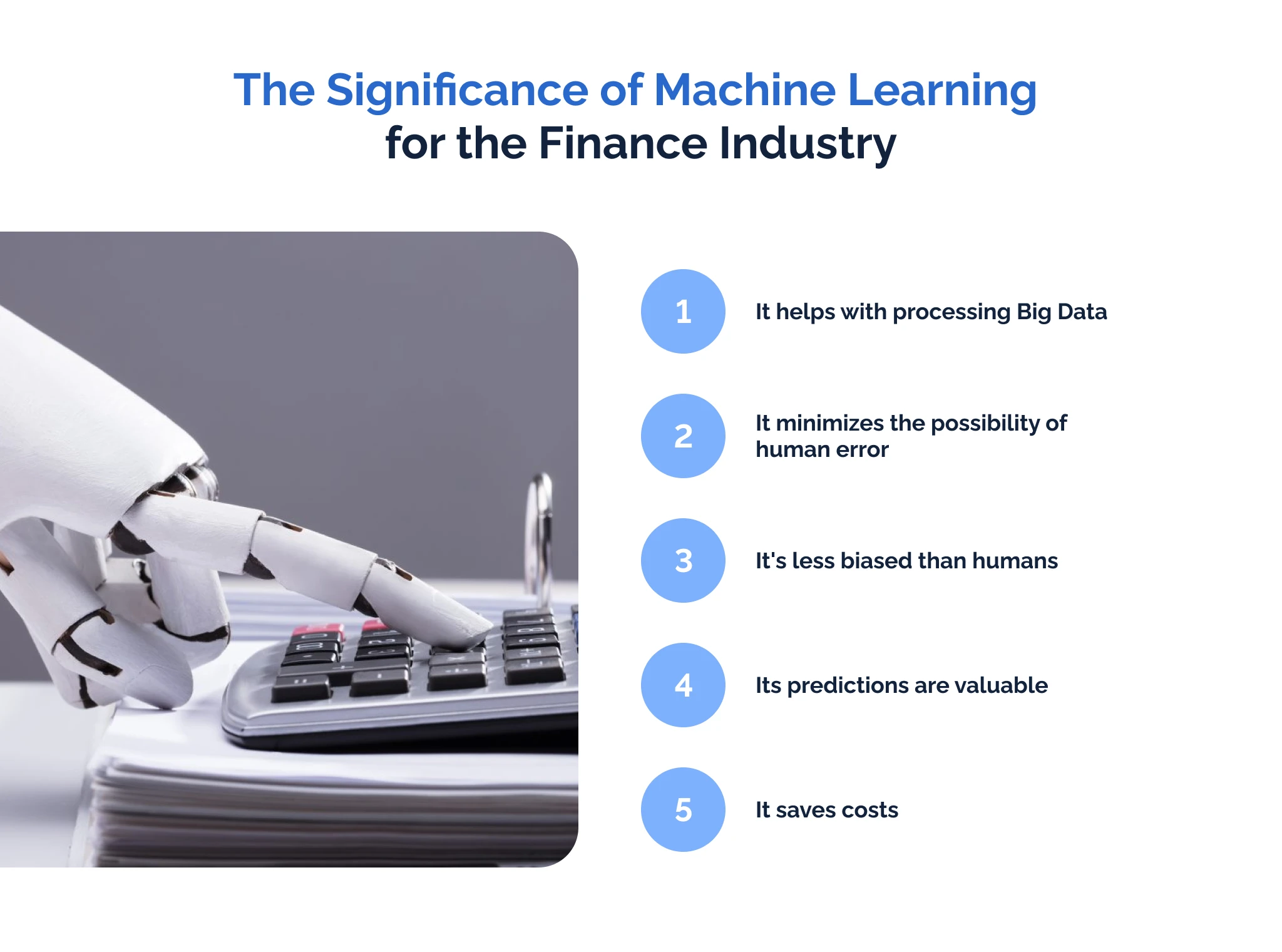
Machine learning in finance is an innovation likely to be industry-defining for many companies. What are the key reasons for making this assertion? Here are the key factors:
It helps with processing Big Data
As we’ve mentioned before, machine learning in finance is of interest because it facilitates the analysis of the so-called Big Data. Big data refers to large volumes of complex and diverse information that can’t be easily managed or analyzed using traditional methods, requiring specialized tools and techniques to extract valuable insights. Automatic neural networks enable us to study this data. They process tremendous amounts of data without getting tired or bored. This approach enables banks to get vital information about their clients and the financial trends that would have been otherwise inaccessible.
It minimizes the possibility of human error
A major problem for many banking organizations is that their workers sometimes make mistakes leading to financial losses (in 65% of all the relevant cases), according to organizations such as Netwrix. Machine learning helps modern companies alleviate the presented problem. How? Firstly, a machine learning tool assists during the process of filling in the data. Using open information from various databases, the tool can highlight the mistakes in the actions of the accountants, for instance. Secondly, machine learning in finance helps with learning about various discrepancies in financial data. It’s a perfect tool for finding information about money laundering or understanding if there were some large-scale errors in the existing bonus programs.
It’s less biased than humans
Machine learning in financial services offers an opportunity to reduce the biased nature of the modern bank system. Why? If given proper instructions, machines can work according to a framework treating all people as equal without introducing any ‘personal opinion’ or resistance. The skeptics can say that machines may reflect some biases of their creators. They, after all, rely on the information humans produce for creating content. What’s the problem with this argument? If one feeds non-biased information to a machine, the problem resolves itself. Machine learning can become a perfect tool for proliferating biases, as TechTarget, a major technology and marketing site in the U.S. notes, but it also provides a pathway to enforcing equality-centric frameworks strictly.
Its predictions are valuable
A significant reason to consider investments in machine learning in finance is the value of predictions. With companies investing in market analysis, they gradually collect the ‘low-hanging fruits’ regarding the optimization of their processes. Using the data accessible for analysis to humans, they resolve some major problems in their functioning. What’s the problem with this approach? No solutions are likely to be perfect. One can add more and more data to the analytical model, changing the seemingly obvious conclusions made by humans. In this respect, machine learning is an essential technology. It offers conclusions disrupting the limited logic of human decisions. Such insights are valuable because they enable companies to go for revolutionary changes in their functioning.
It saves costs
Machine learning in finance reduces the need for labor of many types. Using automatic tools, one can analyze data or provide advice to customers. A neobank, for instance, minimizes costs regarding the analysis of credit histories. Via a machine learning tool, it can calculate some of the key risks of offering a loan to particular people. This approach helps save major sums of money regarding everyday financial activities.
Related services
BANKING AND FINANCIAL SOFTWARE DEVELOPMENT SERVICES
The Most Important Benefits of Machine Learning in Finance
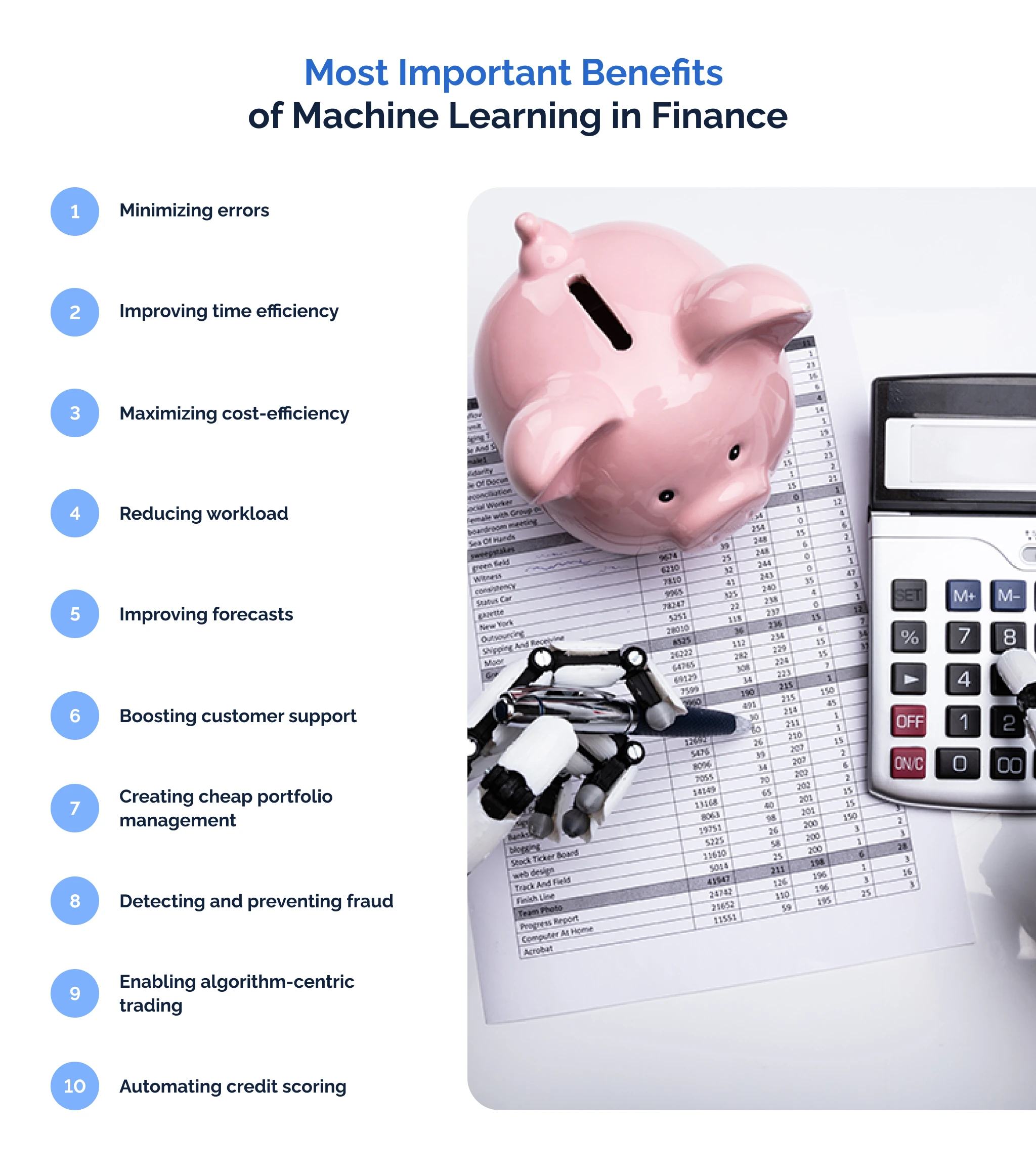
Are you interested in trying machine learning in finance? Here are the key benefits you can get from taking this decision:
Error minimization
Machine learning offers a strong tool for preventing errors. Using it, one can double-check all human decisions to ensure they don’t contain irrational choices. AIs, which are a product of machine learning, are a perfect tool for reviewing all key financial decisions in a company.
Improving time efficiency
Some of the traditional banking practices are slow. For example, taking out a loan is a long process because it requires credit history analysis and major paperwork. Machine learning tools help automate the presented processes. Such frameworks allow modern neobanks to give out loans rapidly and without long confirmations. Analysis of financial data is also a shorter process with the use of machine learning. Using modern high-speed processors, they can review the information that will take thousands of years for humans to analyze in a matter of hours.
Maximizing cost-efficiency
Machine learning boosts cost efficiency. How? It, for instance, decreases the need for labor regarding customer service and information analysis. This is a perfect opportunity to save funds on many work-related processes. Why spend money on expensive labor if a machine can do many things as efficiently as humans?
Reducing workload
Machine learning in finance is also beneficial for workers. Why? It decreases the workload faced by the individuals. The analysts, for example, no longer need to study all the data personally. Instead, they concentrate on the things that matter: the interpretation of the information they possess. Automation isn’t only about replacing jobs. It’s also about the liberation of labor. According to Joe McKendrick writing for Forbes, it helps make work more enjoyable than in the past.
Improving forecasts
Forecasts of any type require in-depth information about certain phenomena. Why is machine learning in banking and finance so important in this regard? It offers access to Big Data. With access to a greater scope of information, analysts can make better predictions regarding the world.
Boosting customer support
What’s the problem with traditional customer support systems? It’s easy to overwhelm them. Maintaining a support system that offers answers to all customers fast is difficult. Responsive support systems tend to be extremely expensive. They’re de facto a major luxury for the companies using those frameworks. In this regard, machine learning in finance is a solution. How exactly? Using AI tools that have a direct relation to machine learning, machines can learn to generate answers to the questions of customers. This approach limits the need for human operators to help the customers.
Creating cheap portfolio management
Having a portfolio manager is an expensive service. In reality, many of their activities are easy to automate. Machine learning tools help users create portfolios without the significant involvement of human professionals. These platforms analyze the data automatically and give advice.
Detecting and preventing fraud
Many people use financial systems to launder money or deceive others. Those activities tend to have clear markers. Machine learning for finance improves the chances of discovering such challenges early on. Within minutes, they can analyze the amount of data that would push humans to the limit of their capabilities for several days. This approach allows for lowering the negative tendencies regarding the financial sector.
Enabling algorithm-centric trading
Trading in the stock exchange often requires a long-term strategy rather than immediate actions. A person may have short-term losses due to their investments, but the approach to investment can lead to long-term profits. What’s the problem with trading? It requires personal presence in many cases to adjust the relevant strategy. Machine learning for finance resolves this problem. One can provide a strategy to a machine, and it will use algorithms to make rational decisions without any other human input. This is a perfect framework for combining trading with other types of daily work.
Automating credit scoring
Machine learning tools help automate the credit scoring process and improve its quality. Firstly, they lower the need for human labor regarding financial assessments. Secondly, they’re better than traditional algorithms due to their ability to analyze non-conventional cases. All this makes it possible to automate the process of assigning credit scores to particular cases.
Challenges of Machine Learning in Finance
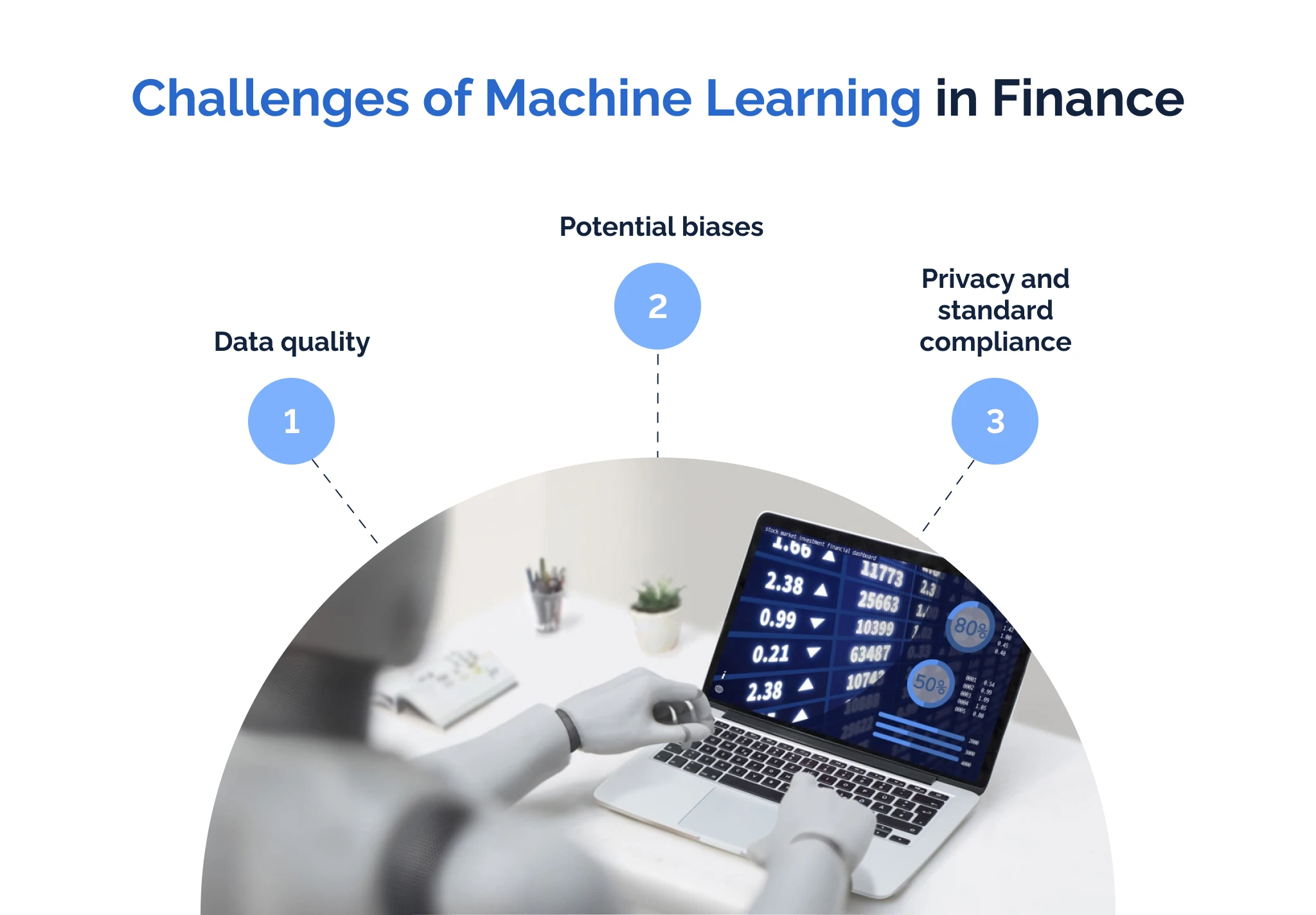
Data Quality
The first significant problem of machine learning is data quality. Yes, MT in finance can boost the speed of data analysis. The problem is with the data itself. There’s a famous expression in mathematics: “garbage in, garbage out.” The quality of input defines the quality of the output. The people providing the data can be lying (intentionally or unintentionally). To facilitate good analysis, one needs something beyond machine learning: it’s also crucial to have strict control of the data.
Potential biases
The problem with machine learning frameworks is that they tend to build complex systems of analysis. Analyzing all their aspects is a long process: we can miss some negative tendencies (for instance, racist ones) in the presented frameworks. In this light, there’s a danger a system becomes biased. This can happen if it processes biased information its creators produce. One should always subject the decisions of a machine-learning system to human analysis.
Privacy and standard compliance
The final challenge in machine learning development is that it can involve private information. Many banks and other analytical organizations claim that they use non-identifiable data. In reality, scientists clearly show such information can be potentially used to identify some people. This factor leads to data leaks and potential prosecution of businesses on the part of the state via the existing privacy-centric laws. If you want to invest in finance and machine learning, you have to ensure your system respects the privacy rights of the clients.
Machine Learning in Finance: Helpful Use Cases
What are some use cases for machine learning in finance? Let’s take a look:
1) Fraud Detection: Machine learning in banking and finance can help with analyzing large volumes of transactional data in real time, identifying patterns and anomalies to detect fraudulent activities, preventing financial losses, and protecting customers.
2) Credit Scoring: By analyzing historical data, machine learning models can assess creditworthiness more accurately, enabling lenders to make better-informed decisions and offer loans with appropriate terms while reducing default risks.
3) Algorithmic Trading: Machine learning algorithms can analyze market data, identify patterns, and make rapid trading decisions, optimizing investment portfolios, reducing human bias, and potentially enhancing profitability.
4) Risk Management: Machine learning apps in finance can help quantify and manage financial risks by predicting market movements, identifying potential vulnerabilities, and optimizing risk-reward trade-offs. This enables financial institutions to make informed decisions and mitigate risks more effectively.
Future of Machine Learning in Finance and Banking
What are the long-term outcomes of bringing machine learning in finance from theory to practice? Firstly, it offers great opportunities for personalization. By analyzing individual data, you can create unique propositions for the bank customers, for example. This is a perfect way to boost client loyalty. Secondly, machine learning boosts anti-fraud defenses. AIs can learn to detect various types of fraud and even discover new ones. This factor is crucial for boosting customer security. Lastly, we believe machine learning gives an opportunity to create automated customer support. Support apps based on models such as ChatGPT can offer an opportunity to minimize labor in the sphere of customer support. Machines that know how to answer complex prompts will help resolve many complex situations related to bank services.
Conclusion
To summarize, the presented information indicates machine learning in banking is a worthwhile investment. It offers an opportunity to introduce new services for the customers and decreases many risks. If you’re interested in investing within this sphere, we recommend looking for professional companies with experience in the financial field. We at Keenethics, for instance, have experience developing multiple projects in the financial sector.
FAQs
How do people use machine learning in finance?
People use machine learning in finance to automate tasks, analyze large amounts of data, make predictions, optimize portfolios, and manage risks.
What algorithms are crucial for machine learning in finance?
Crucial algorithms for machine learning in finance include linear regression, decision trees, random forests, support vector machines, neural networks, and deep learning models.
Can ChatGPT be used in finance?
Yes, ChatGPT can be used in finance. It assists with answering finance-related queries, providing information on financial products, explaining concepts, offering investment insights (not as financial advice), and discussing general financial topics. Still, one should be careful regarding this technology as it occasionally tends to offer incorrect information.
Keenethics can offer you consultations on this issue and assist in developing a high-quality product.