This article discusses the use of machine learning in agriculture. Learn how to use cutting-edge technologies in this blog post!
The core goal of all agricultural companies is to raise their profits. One of the best ways to do this is to increase crop yields and, more importantly, their simultaneous quality. In this article, we’ll look at the technology that can simplify the attainment of this goal. This technology is machine learning for agriculture. Modern AI innovations that appear in software for the agriculture industry are, ultimately, one of the best ways to raise the productivity of your farms.
What Does Machine Learning Stand For?
Machine learning is a technology that has applications in a wide variety of fields. Its essence is simple: the creators of machine learning frameworks mimic the neurological structure of the human brain and then train the artificial networks on vast arrays of data. For example, machine learning algorithms are behind the recent rise of Large Language Models. The creators of those models trained their frameworks on large corpora of human texts. These corpora, in turn, allowed them to achieve great accuracy in terms of predicting human languages. Ultimately, this seemingly simple trick is behind the staggering complexity of the technology in question. Similarly, it can be used in many fields. For instance, one can use the technology to analyze crop health tendencies in multiple farms. Then, it’ll be possible to clearly understand what goes well and what doesn’t go well on the basis of preliminary information.
What’s the Importance of Machine Learning in Agriculture?
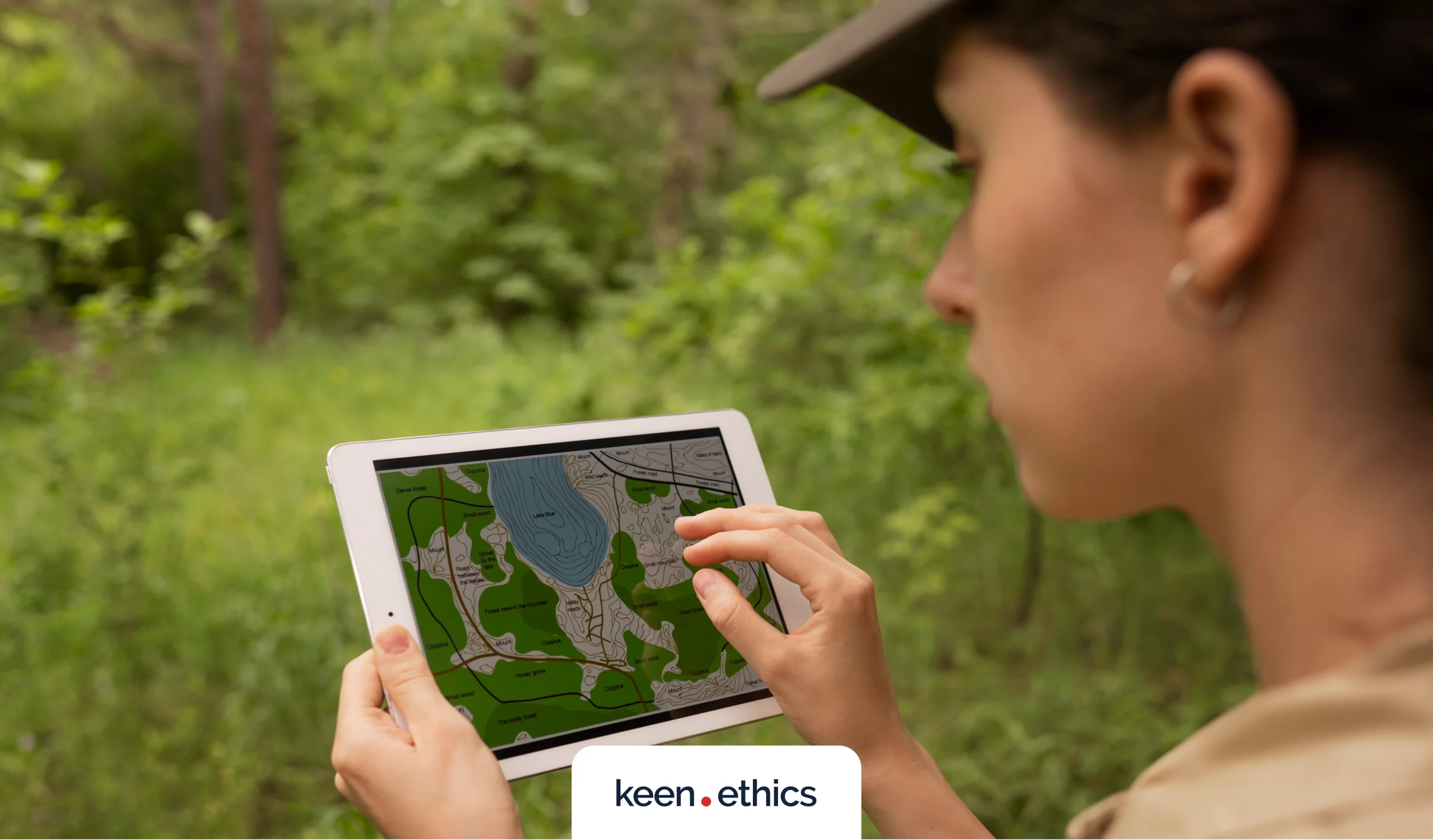
Machine learning in agriculture is going to have many core applications. Firstly, it’ll be possible to apply this technology in the field we’ve mentioned above. Many companies will be using machine learning to analyze crop health in their business. Secondly, this technology is also likely to have a wide set of applications concerning smart farming. In this regard, machine learning tools connected to sensors will be able to analyze soil types and then adjust irrigation and even temperature (in the case of greenhouses) to the diverging needs of the relevant farms.
More importantly, the technology in question goes beyond simple algorithmic automation. The problem with traditional algorithms is that they don’t have any capacity for advanced pattern recognition. They act in similar ways regardless of the involved situations. Consequently, those models are applicable in a relatively narrow set of circumstances. In many ways, machine learning for agriculture offers a solution to this issue. Machine learning tools are capable of acting autonomously based on the data they’ve received in the past. Hence, the technology now offers an opportunity not only for automation but for automation that is smart and adaptable.
Applications of Machine Learning in Agriculture
Let’s look at the agriculture activities that can greatly benefit from the application of machine learning technologies in their everyday work scenarios. The key goal of using this technology is to forgo the traditional methods of management and promote an alternative pathway based on advanced automation.
Detection of malignant plants
Malignant plants like Palmer Amaranth can cause tremendous losses for the majority of agricultural facilities. The problem with such weeds is that they can start growing in an unexpected fashion. As a result, you’ll be able to detect them only after the damage is already done. This happens because the majority of fields are large and require multiple individuals to guarantee at least a partial understanding of their real state. In this light, modern machine learning tools coupled with IoT devices can find traces of malignant plants early on. Using this data, the neural networks will quickly inform you about the potential problems, enabling quick removal of unwanted plants. In many cases, even small changes to irrigation schedules can guarantee a difference between success and failure.
Detection of crop diseases
Diseases are one more problem that affects many farms in the modern world. Once again, the average farm faces a major problem with their detection in the early stages. Human observers are likely to see major issues only once they become advanced enough. Therefore, machine learning in agriculture finally offers a long-term solution to the problem. Armed with sensors, diverging frameworks in modern agriculture can quickly detect anomalies before they become widespread. Diseases belong to this type of anomaly; hence, you can quickly detect them with the help of prediction-oriented machine-learning tools.
Prediction of yield levels
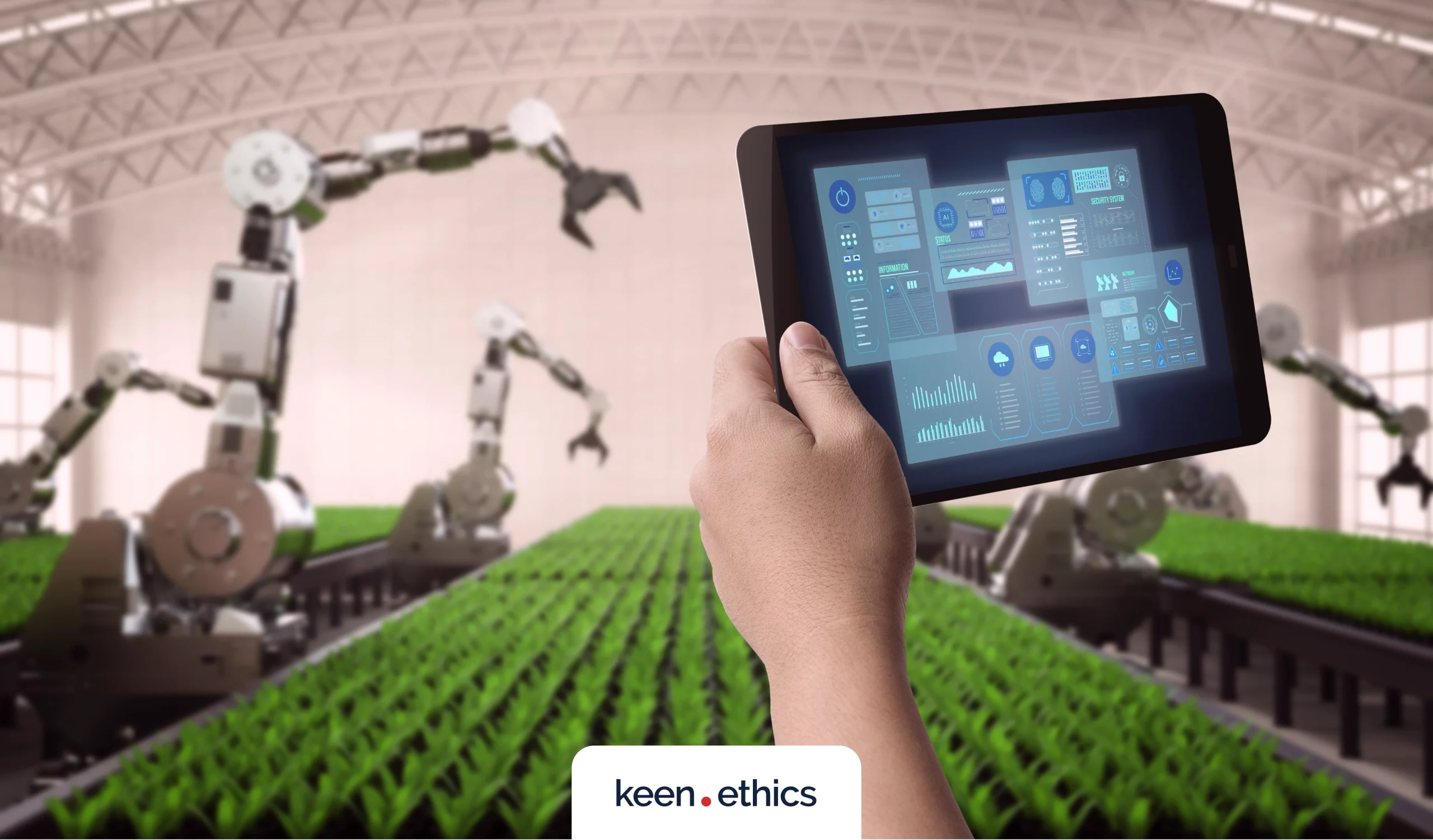
One more problem with agriculture is that you never have a full understanding of your upcoming yields. In this regard, the ability or inability to predict yields may have a decisive impact on the price of your wares. For instance, a lack of yield predictions can end in low-quality agreements with partners due to lower or higher yields than expected. Deep learning algorithms are notable for their ability to analyze yields in thousands of fields. More importantly, this advanced technology is also capable of analyzing your field, too. Using this vital information, machine learning tools should be more than sufficient to predict your agricultural production based on the relevant inputs you and the installed sensors offer.
Breeding assistance
Modern deep-learning algorithms are also vital for diverging types of breeding. They can affect livestock production by helping you select the most positive traits in your livestock. Usually, selection is done via apps that primarily focus on recording or, at best, algorithmic predictions. Machine learning tools, however, can go beyond algorithms and offer you more advanced predictions. For example, it’s possible to analyze the lineages of several animals to offer predictions on the manifestation of particular traits. No other tools are capable of offering predictions of this accuracy apart from machine learning in agriculture.
Irrigation assistance
Irrigation is among the most vital aspects of agriculture in the modern world. If you don’t offer a balanced amount of water to your plants, they’ll inevitably fail to provide sufficient yields. Modern machine learning tools are notable for a solution to this problem. So, what do they do? Firstly, these instruments have the in-built capacity for crop recognition. This means that they can deduce which crops are being grown, adjusting their irrigation frameworks to the needs of your farm.
Secondly, they also have a capacity for crop monitoring with the help of various sensors. Based on this crop monitoring, it’s possible to adjust irrigation in ways that will optimize yields. Generally, the relevant tools are capable of taking dozens and even hundreds of factors into consideration and then reacting to them in unique ways. A traditional algorithm system may have no adaptations for issues such as crop diseases. In turn, tools like machine learning can quickly learn how to adapt to those problems in real time.
Malfunction detection
Malfunctions in various production systems aren’t an uncommon problem for large farms. For instance, an irrigation pipe may encounter leaks that have the potential to be undetected for a long time. Those problems are capable of ultimately resulting in major issues with crop yields. Machine learning algorithms that are helped by various sensors offer a solution to this problem. They can detect problems based on additional data from your farm. Therefore, the assistance of machine learning tools is among the best ways to prevent unnecessary losses on your farm. Here, it’s also easy to see one of the core advantages of machine learning models. What’s this advantage? This advantage is the capability of the tools in question to predict certain events based on more or less incomplete data.
Tools for monitoring weather
Bad weather is still a major negative factor for the majority of farms. For example, a drought may easily result in greatly diminished yields. The core goal of machine learning in agriculture is to help you with predicting various negative events before they occur based on secondary data. In this case, modern AI machine learning tools are also capable of offering significant long-term positives for the farmers. Your algorithms can connect to weather station reports and then use data from, for example, 100 years of observations to offer predictions on future trends in the upcoming months. Situations like droughts are relatively easy to predict if you know a myriad of factors causing them. Humans typically don’t have enough processing capacity to analyze every factor. Tools for machine learning in agriculture, however, should be more than sufficient to analyze tremendous amounts of data and then offer realistic long-term predictions.
Fertilizer optimizations
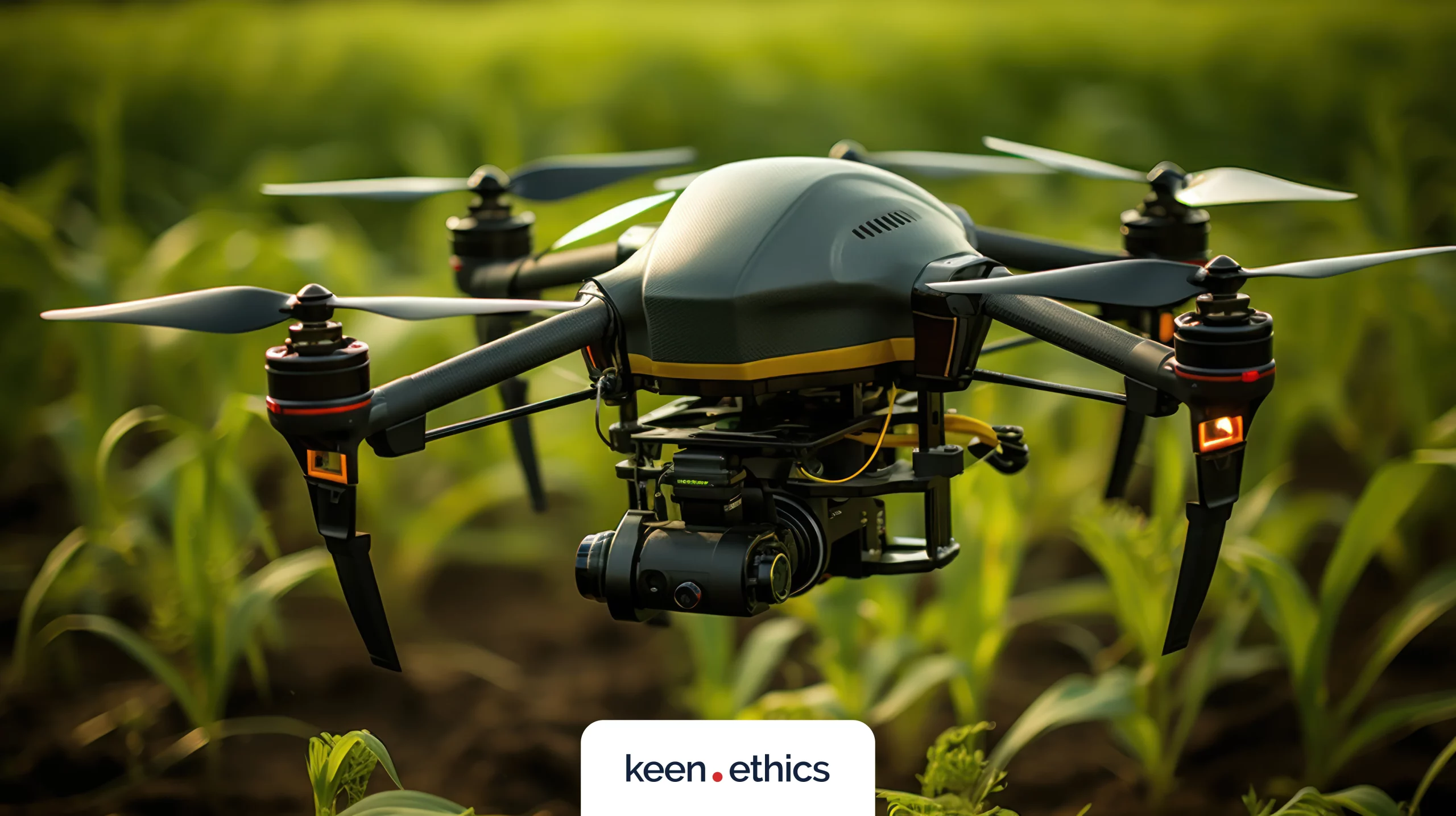
Fertilizers are a technology that is behind a tremendous growth in the productivity of agriculture. However, there’s one obstacle that leads to skepticism concerning this technology. Fertilizers are known for being potentially toxic if used in large doses. Together with pesticides, they’re more than capable of depleting soils across the world if used incorrectly. Machine learning in agriculture once again solves the problem of overusing or underusing fertilizers. In this regard, one can build an advanced model of agriculture activities with the aid of machine learning. Using those models, it’ll be relatively easy to predict whether the usage of certain fertilizers is optimal or suboptimal in particular use cases.
Instruments for monitoring soil
There are many tools for monitoring soils available on the market. The problem is that they have to be applied manually, making long-term observations a difficult approach. The combination of IoT and machine learning finally solves this problem for modern farms. How exactly do these technologies achieve the goal in question? Above all, by constantly analyzing information from sensors and then transforming it into long-term prediction frameworks. With the help of those tools, it’s possible to know which plant is optimal for your soil and which isn’t.
Frameworks for detecting insects
Insects represent a major long-term problem for the majority of farms. An insect swarm can easily destroy the crop yields for many years to come. Machine learning tools help with this, too. Firstly, you can use those tools to analyze environmental data from multiple locations and predict whether some insect swarm would affect your farm negatively in the first place. Secondly, those tools are also perfect for detecting whether insects appear on your farm at all. Lastly, various robots under the control of insect detection tools may be sufficient for the dispersion of anti-insect substances. Furthermore, it may even be possible to combat the problem automatically with the help of this technology.
Detection of diseases in animals
Diseases in animals are one more problem that can be devastating for modern farms. Some diseases simply result in losses among the livestock. Others, however, may have devastating impacts during consumption, like CJD, which affected some beef consumers in the UK during the year 2000. Machine learning in agriculture helps with detecting such problems early on by, for instance, reviewing the sporadic behavior of the involved animals. In the end, the technology offers improved safety for the consumers and, more importantly, enables one to maintain high yields without contamination.
Feed monitoring
Feeding is an essential component in the process of raising animals. Insufficient feed may result in long-term difficulties with their health. The outcome is the disruption of meat or milk yields on a farm. Modern software in the sphere of machine learning presents a solution capable of resolving this problem. You can fully automate feeding with the help of sensors and dispenser systems. More importantly, every aspect of feeding (including animal weight) will be monitored by the relevant machine-learning frameworks to prevent any long-term problems with various machine malfunctions. Machine learning algorithms don’t only automate feeding but also know when problems with water or feed supply occur in the first place.
Tools for controlling grazing
Lastly, the market of machine learning instruments also includes many tools for controlling grazing in a farm scenario. The relevant machine-learning tools are necessary for selecting the optimal feed regimen for your animals. Moreover, they should be sufficient for finding the most appropriate fields. Once again, machine learning tools know how to collect high-quality insights. As a result, they’re the most optimal frameworks for selecting the best fields aimed at maximizing the potential of your ranch.
Key Benefits of Machine Learning for Agriculture
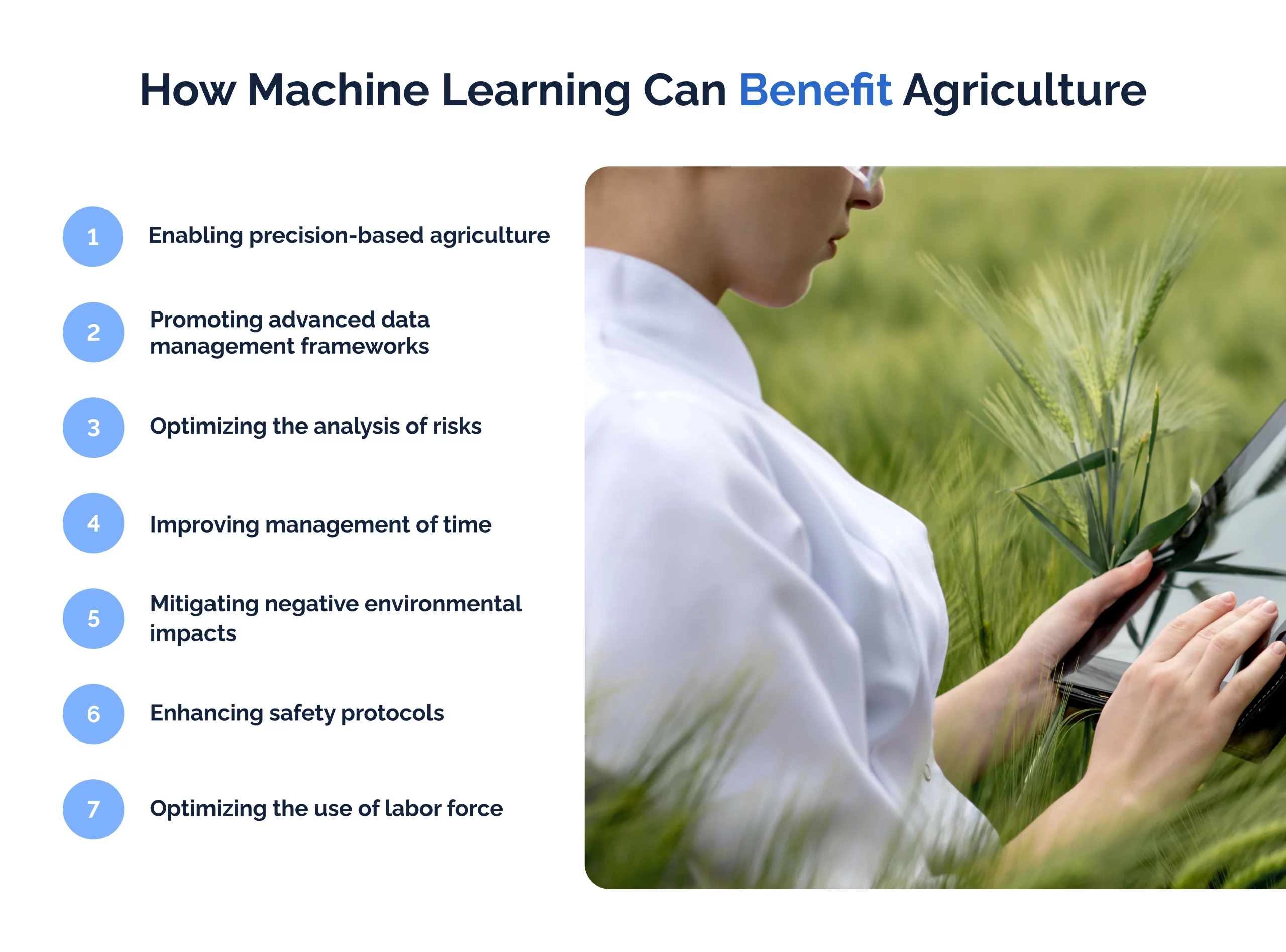
Machine learning offers a wide set of benefits for the modern agricultural sectors. Here’s a list of the core positives you can get through the use of this technology.
Precision-based approach to agriculture
In the past, agriculture had to depend on the relatively inaccurate assessments of the farmers. The appearance of machine learning tools enables a novel, precision-based framework. For instance, you can optimize the use of resources through the analysis of weather patterns. It’s also possible to have complete predictions of the upcoming yields. Big data and machine learning tools become essential for making advanced predictions in the sphere of agriculture.
Advanced frameworks for data management
Data management is one more aspect that greatly benefits from machine learning in agriculture. If connected to proper sensor tools, the relevant systems can collect tremendous amounts of data and then transform it into vital insights through aspects like regression analysis. The ability to work with tremendous amounts of data and then manage it is the core benefit of modern machine learning tools.
Improved risk management
There’s also an opportunity to improve risk management with the help of the relevant tools. Many factors such as temperature can have long-term negative impacts on your animals or crops. Machine learning tools are capable of creating elaborate scenarios based on different inputs. Therefore, they should be more than sufficient for analyzing potential threats to your operations. The more data you offer to the frameworks in question, the better.
Upgraded management of time-limited scenarios
In many situations, you work with rather tight deadlines within the agricultural field. This information means that there’s always a risk of skipping some important data. For instance, if you don’t take some images of a field on time, the lack of information may end in the manifestation of negative traits. Machine learning in agriculture provides users with a wide range of tools for automating those processes. As a result, there’s a much lower probability of making some major mistakes.
Minimized environmental impacts
Modern machine learning tools are primarily noteworthy for their ability to analyze certain situations and then make predictions regarding them. Machine learning in agriculture is notable for its ability to predict cases in which negative environmental impacts manifest in various ways. Consequently, there’s always a chance to minimize the potential negative impacts on your farm.
Improved safety for workers
A big problem in the agricultural field is the safety of the workers. Certain activities in it are potentially hazardous. Machine learning tools are capable of controlling certain machines in an automatic mode. Thus, there’s always an opportunity to automate your farm through a data-driven approach. Machine learning in agriculture is a perfect way to capitalize on this capability of the technology in question.
Labor-saving benefits
Machine learning tools are among the best frameworks for automating tasks like the analysis and monitoring of livestock density. This information means that ML in agriculture is a great pathway for reducing the reliance on human workers in the management of a farm. This technology is a definitive labor-saving tool. As a result, it’s almost certain to end in a significant rearrangement of the workforce scenarios in modern agriculture.
How to Solve Agricultural Problems Through ML Technologies: A Hypothetical Example
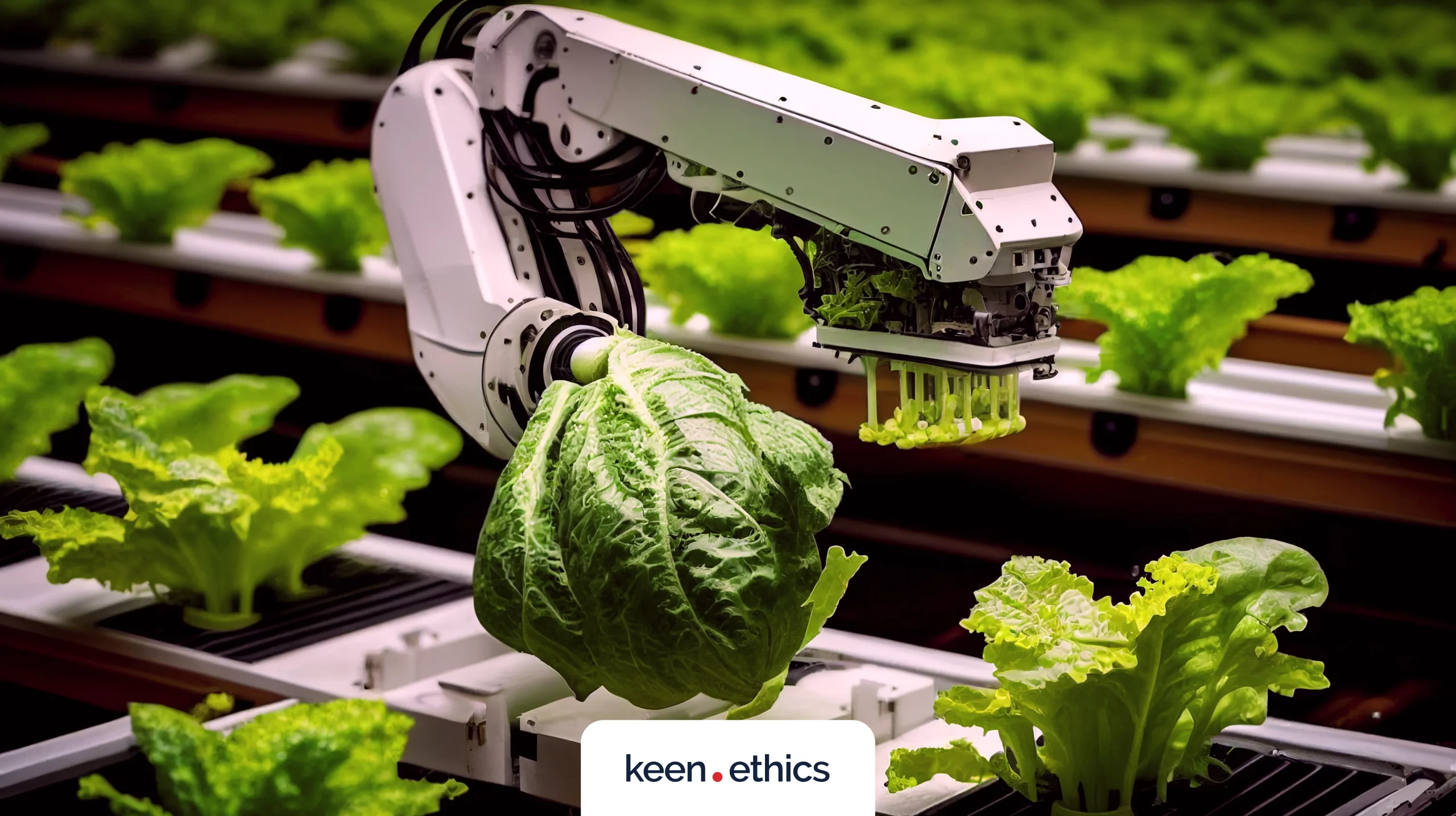
Let’s imagine that you have a farm in a high-risk agricultural zone. Your yields may greatly depend on the weather conditions in your region. ML in agriculture is a technology that’s capable of resolving your core problems. Through imaging processing technology, for example, an ML framework can analyze zones that need your utmost attention. More importantly, weather predictions can help you with adjusting irrigation to mitigate the impacts of bad weather on your agricultural products. Consequently, the usage of algorithms may be essential for raising the long-term yields of your business.
What’s the Future of Machine Learning Tools in Agriculture?
Machine learning tools in agriculture are definitely going to have a rather positive future. Above all, we expect improvements in the complexity of the machine learning tools. Our algorithms for machine learning are becoming more complex. Thus, it’s reasonable to expect that they’ll produce increasingly advanced behaviors. The advancement of this technology will also end in generally lower prices for its adoption. It’s becoming easier and easier to use tools of this kind these days.
Conclusion
To summarize, the presented information indicates that investments in machine learning tools are a highly rational decision. These tools can genuinely transform your business and add many positive characteristics to it. Consequently, the earlier you adopt various machine learning tools, the better. It’s reasonable to be swift about those changes: the growth of sectors like IoT, which is gaining 12.6% scope per year, indicates that most companies on the market are investing in technologies of this kind.
Keenethics is capable of helping with their adoption!